The AI shifts that will shape 2025
For years now, AI conversations have bounced between two extremes: utopian promises of limitless potential and doomsday warnings of an uncontrolled future. But in 2025, we’re past the speculation phase. AI is no longer a concept to be debated; it is a business function, an operational tool and a market differentiator.
Despite its growing footprint, AI is still widely misunderstood. Adoption isn’t the same as impact and many organisations remain stuck in a loop of experimentation without ever unlocking real value. This year, the most important AI shifts aren’t just about the technology itself but also about how businesses utilise it.
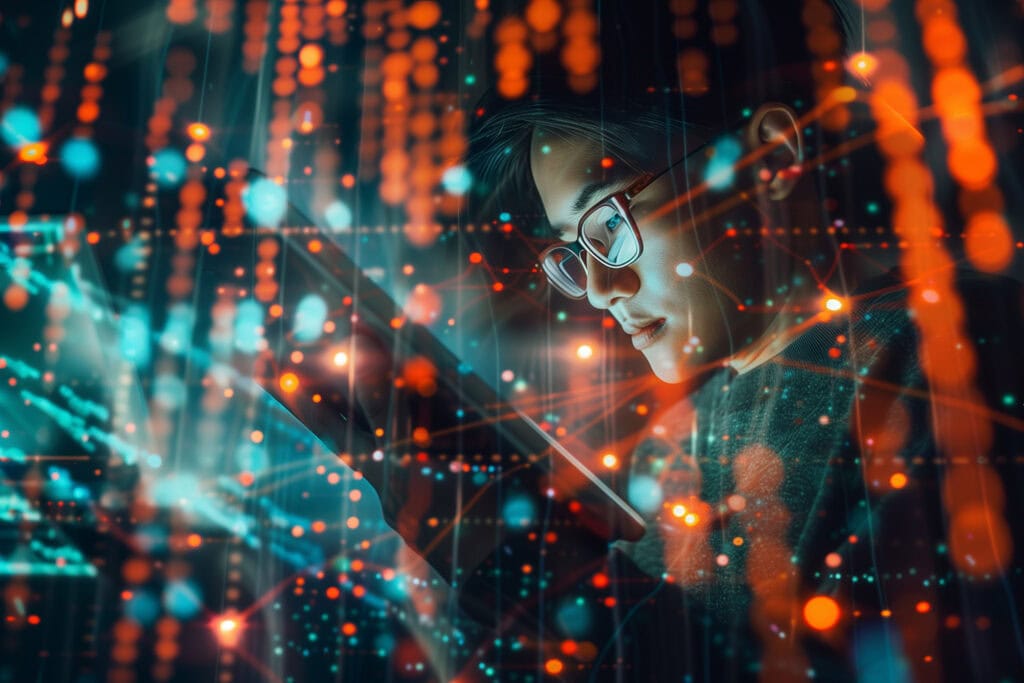
1. AI governance and regulation catch up
With AI integration accelerating, Guardrails, Ethics, Regulation and Governance (GRC) is no longer a ‘nice to have’ – it will soon become a necessity. In 2025, organisations will be under greater scrutiny to implement AI guardrails that monitor inputs, outputs and interactions. The EU AI Act, the first ever comprehensive AI legislation, will begin enforcement in August 2025, setting a precedent for global AI governance. Meanwhile, countries around the world are drafting their own regulations, meaning AI compliance is about to become as important as cybersecurity and financial reporting. Beyond regulation, trust and transparency are now key differentiators. AI audits, governance frameworks and “model cards” that explain how AI makes decisions will become standard. Businesses that proactively build AI accountability into their operations will not only avoid regulatory risks, but will also earn stakeholder trust.2. Maturity in AI evaluation
As AI becomes a core component of business strategy, the next challenge is not just adoption, but evaluation. Organisations are moving beyond proof-of-concept and asking a critical question: is AI working as intended? AI agents are transforming business automation, shifting from rigid workflows to adaptive systems that make decisions and interact dynamically with other tools. Unlike traditional AI models, these agents don’t just process information, they orchestrate multiple technologies, assess real-time data and adjust autonomously. This adaptability introduces new challenges in evaluation. Traditional AI metrics don’t fully capture how well an AI agent performs across different tasks and decision points. Businesses need a broader evaluation framework; one that moves beyond efficiency to assess how AI integrates with human teams, navigates complexity and ensures reliable, real-time decision-making at scale.3. Small language models (SLMs) prove size doesn’t always matter
In 2025, AI won’t be about using the biggest model, it will be about using the right model.While Large Language Models (LLMs) have driven AI advancements, SLMs are becoming the smarter choice for many businesses. SLMs are built for specific tasks, making them faster, more affordable and easier to manage. Unlike LLMs, which require significant computing power, SLMs can operate within a business’s existing systems, reducing cloud costs and improving response times. This makes them especially useful for industries using AI on the ground, like robotics, drones and IoT. For companies, this shift means more flexibility. Many business needs can be met with these smaller, tailored models, offering reliable accuracy without the high costs or complexity of larger AI systems.4. AI “Super Platforms” are set to reshape the industry
AI startups have long been at the forefront of innovation, driving breakthroughs in machine learning, automation and specialised AI applications. But in 2025, we’re seeing a shift. As the development of Generative AI applications surges, businesses are encountering overlapping capabilities, prompting a move toward consolidation. Agile disruptors are being absorbed, concentrating AI talent and technology under a handful of dominant players. This shift is creating AI super platforms, aka end-to-end tech ecosystems that integrate cloud computing, pre-trained models and automation tools in one place. For businesses, this means greater access to integrated AI solutions but an increased reliance on just a few providers. With major players shaping AI’s development, organisations must carefully consider who controls their AI infrastructure, how flexible their AI solutions are and what dependencies they may be locking into.5. The rise of multimodal AI and the path toward greater intelligence
Multimodal AI reasoning, aka models that process, generate, and reason across text, images, video and speech, is becoming more advanced and adaptable. This shift is already transforming industries, from robotic assistants that can see and respond to human interaction to AI-powered analytics that merge satellite imagery with real-time data for climate and agriculture insights. Meanwhile, discussions around Artificial General Intelligence (AGI), which refers to AI capable of human-like reasoning and adaptability across diverse tasks, are gaining momentum. While AGI remains theoretical, Artificial Superintelligence (ASI) is an even more distant concept, referring to AI that surpasses human intelligence entirely. However, businesses shouldn’t focus too much on these future advancements. Instead, the priority should be on adopting and maximising current AI capabilities today. Multimodal AI is already delivering efficiency and innovation, but its long-term potential will depend on how organisations integrate and govern these systems.6. The evolving complexity of AI inference
Training AI models are just the beginning. The real challenge is making them work efficiently in real-world applications. In 2025, AI inference, aka the process of running models on live data, is becoming a critical focus as businesses seek to improve efficiency, accuracy and governance. As AI adoption scales, businesses are refining AI Operations (AIOps) frameworks, including Machine Learning Operations (MLOps), Foundational Model Operations (FMOps) and Large Language Model Operations (LLMOps). These ensure AI systems remain efficient, explainable and compliant. Optimising inference is key for industries requiring real-time AI decisions, such as robotics, autonomous systems and AR/VR. Advancements in model compression, accelerated inference and edge computing are reducing cloud dependence, improving speed and lowering costs. Two forces are driving this shift:- Scalability and cost – As AI models grow, inference efficiency is as essential as training.
- Regulatory and privacy pressures – Data laws (Australia’s Privacy Act 1988, the Consumer Data Right (CDR) and international regulations like GDPR) are pushing AI processing closer to users.
In 2025, the businesses that refine AI inference will transform it from a technical challenge into a tangible driver of efficiency and growth.
The key takeaway? AI at scale is now a competitive advantage.
AI is no longer a future ambition or a boardroom talking point; it’s now a fundamental business capability. But as adoption accelerates, the divide between those who experiment with AI and those who embed it strategically is widening. The real challenge in 2025 won’t be whether companies are using AI, but whether they’re using it well.
At ai decisions, we help organisations move beyond pilot projects, designing and deploying custom AI models and AI agents that are operationally viable, risk-aware and built for real impact. AI is more than a tool; it’s an enabler for smarter decision-making, efficiency and growth.
Follow us on LinkedIn and stay up to date with the latest AI insights and innovations.
About the authors
Adam Kubany holds a PhD. in AI and has over 20 years of experience in IT. He specialises in leading and coaching research and development (R&D) teams to tackle complex Artificial Intelligence (AI) and Machine Learning (ML) challenges with responsibility. Want to chat with Adam? Email adam@theoc.ai
Sridhar Kannam is a seasoned AI professional with 15+ years of experience in developing data-driven solutions to complex business challenges. With a PhD in Computational Science, Sridhar brings deep technical expertise and thought leadership. Want to chat with Sridhar? Email sri@theoc.ai
Authors
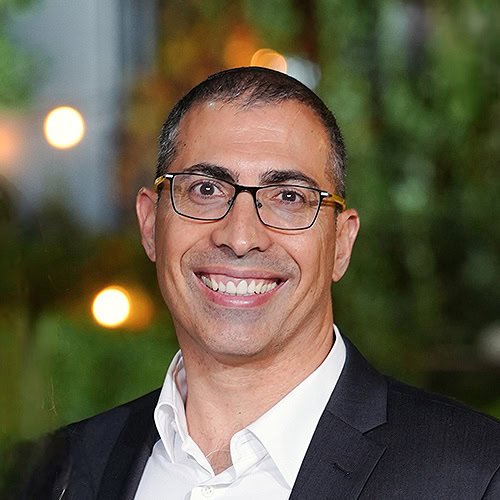
Adam Kubany, Ph.D
Chief Data Scientist at ai decisions
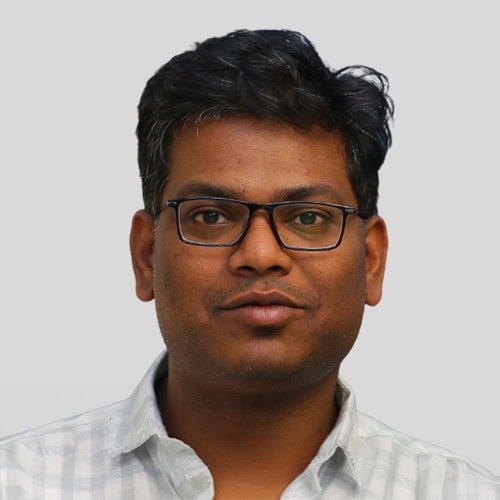
Sridhar Kumar Kannam, Ph.D
Senior Manager – AI/ML at ai decisions