AI risk: who pays the price when it fails?
Artificial intelligence is no longer the experimental tool it once was. It’s making decisions that impact people’s health, finances and livelihoods, and yet in many industries, the rules governing AI remain ambiguous.
That is changing fast. The EU AI Act, set to be enforced in August 2025, is the first comprehensive AI legislation. It will introduce strict oversight for high-risk AI applications, including healthcare, self-driving cars and legal decision-making. The penalties are significant; up to €35 million or 7% of a company’s total global revenue, placing real accountability on organisations deploying AI.
Australia is following suit, with proposed AI safety guidelines designed to close the gap between technological growth and regulatory readiness. While AI-specific legislation isn’t in place yet locally, regulatory oversight is increasing.
In the U.S., the Trump administration recently introduced Executive Order 14179, which sets out new policies on federal agency use of AI and procurement standards. These developments are highly likely to influence Australia’s own regulatory direction.
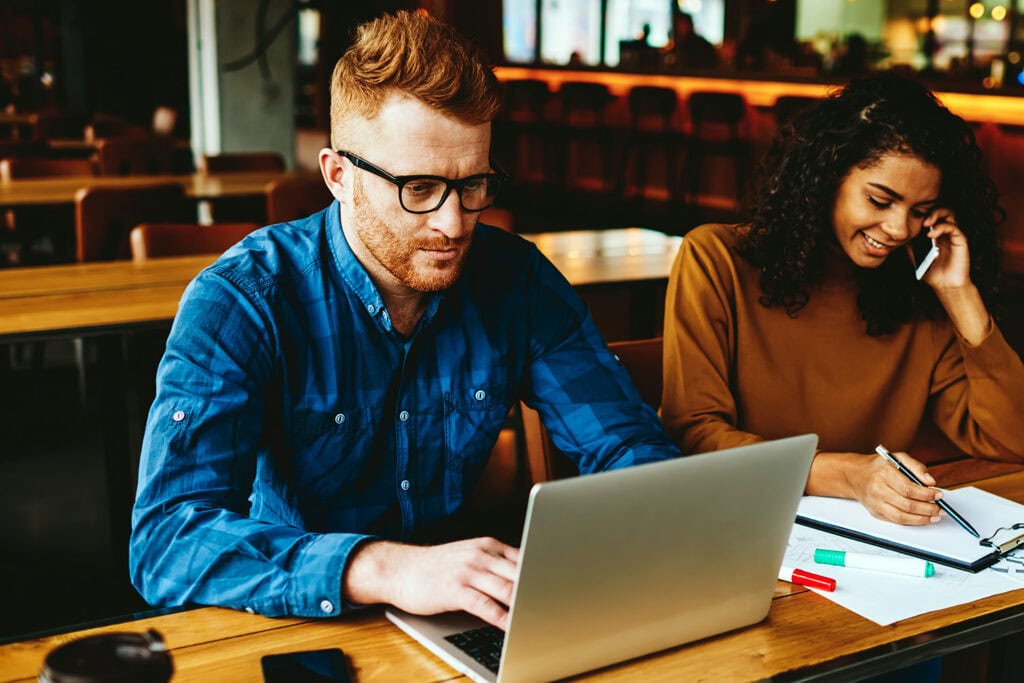
Why not every AI decision is binary
While regulation is beginning to take shape, legislation alone can’t solve the deeper challenge of AI operating in morally complex territory. A well-known ethical dilemma that captures this challenge is the Trolley Problem, introduced by philosopher Philippa Foot in the late 1960s. In this scenario, an out-of-control trolley approaches a fork in the tracks: on one side, five people; on the other, one. It can only be diverted to one path. Who should be saved?
The logical answer might be to save the greater number. But what if the one is a scientist close to curing cancer, or one of the five is a serial killer? Suddenly, the equation isn’t so simple. Can we expect AI to weigh up these kinds of complex moral decisions with the same level of nuance as a human?
Who takes responsibility for AI’s decisions?
AI systems are already making high-stakes calls. When they fail, accountability becomes a pressing concern.
For example, if an AI-powered hiring tool discriminates against a candidate, does responsibility fall on the employer or the AI provider? If a self-driving car miscalculates and causes a fatal accident, is the manufacturer at fault or the AI developer? Or, is it the driver who failed to intervene in time? If an AI system makes a mistake relating to a medical diagnosis which leads to a preventable death, is the liability on the doctor, the hospital, the software company, or the company that developed the pre-trained model in the AI system?
These aren’t theoretical concerns. They’re active legal battles and businesses operating AI systems must be prepared to navigate this complex liability landscape. While the EU AI Act introduces clearer liability measures, Australia and other markets are still playing catch-up.
While the EU AI Act sets out regulatory obligations, a separate EU proposal to clarify liability, the AI Liability Directive, was withdrawn in February 2025. This has created further ambiguity around who is legally responsible when AI systems cause harm. Meanwhile, some jurisdictions are beginning to take a more principles-based approach.
At a state level, the NSW Government has published AI guidance for public servants, highlighting accountability expectations for government agencies. While not legislation, this guidance signals a growing interest in formalising responsibility and suggests that businesses will need to establish their own liability frameworks in the absence of clear global standards.
Without guardrails, AI can cost more than it solves
While self-driving cars once dominated the conversation around AI risk, they’re now just one piece of a much broader landscape. AI is powering decision-making in fraud detection, credit scoring, supply chain logistics, customer service and more. And when things go wrong, the consequences have real-world implications.
Fraud detection models have mistakenly flagged legitimate transactions, locking users out of their accounts and prompting legal disputes. Poorly governed AI in supply chain systems has triggered inventory errors that cost businesses millions. In customer support, AI chatbots have delivered misleading responses, sparking reputational damage.
Despite these scenarios, regulation is still catching up. Frameworks are forming, but they’re often too slow, too broad or not aligned with how AI is actually being deployed. That’s why businesses can’t afford to wait.
Organisations must take the lead in interpreting and operationalising AI governance. That means defining clear roles, embedding oversight and adapting guardrails into internal systems that work in practice, not just on paper.
Responsible AI starts with recognising the risks
Bias and fairness are among the most visible risks in AI, but they’re far from the only ones. As AI systems become embedded in business operations, they also bring newer risks to the surface, such as hallucinated outputs, toxic content, prompt injection attacks, personal data leakage and model poisoning. While the examples may vary, the root issue is the same. AI can behave in ways that are difficult to predict or control.
Take bias as one case. The COMPAS recidivism model used in the U.S. justice system disproportionately flagged Black defendants as high risk, leading to unjust parole denials. That wasn’t accidental; it stemmed from flawed training data and a lack of oversight.
Bias has also appeared in loan approvals, hiring systems and insurance assessments. Toxicity and hallucinations are showing up in customer service, and prompt injection attacks are a growing concern in healthcare and legal applications.
AI governance can’t afford to treat these risks as technical glitches or edge cases. Businesses must take a proactive approach, embedding guardrails from day one.
Correcting AI’s blind spots with Risk Navigator
AI risks accumulate quietly, buried in datasets, model updates, processes and compliance gaps, surfacing as costly failures. Machine learning models degrade over time, meaning performance and accuracy can drop without ongoing evaluation and recalibration. Beyond avoiding AI missteps, embedding governance that enables AI to scale responsibly is key.
Risk Navigator was designed by ai decisions to do exactly that. Built on global best practices, including NIST, ISO, MIT and IBM frameworks and aligned with Australian regulatory bodies such as ASIC, APRA and the Australian AI Ethics Principles, Risk Navigator provides a structured, real-world approach to AI risk management.
Covering every stage of the AI lifecycle, from data collection and model development to deployment and continuous monitoring, Risk Navigator helps organisations detect vulnerabilities early, align AI initiatives with evolving regulations and implement the right safeguards to mitigate risk without stifling innovation.
Turning AI risk into a strategic advantage
Governance shouldn’t be seen as a blocker to AI adoption. In fact, it’s what allows organisations to use AI effectively and with confidence. When built into systems and processes from the start, governance becomes a foundation for trust and long-term value.
Risk Navigator enables organisations to map AI risks to specific compliance obligations, ensuring that governance isn’t a reactive exercise but a proactive enabler of responsible AI deployment.
With AI adoption accelerating across industries, the real risk isn’t in moving too fast, it’s in moving blindly. Now is the time to take control of AI risk before it becomes a problem.
Want to know how Risk Navigator can help your organisation stay ahead of AI regulation? Let’s chat. Email info@theoc.ai
Follow us on LinkedIn and stay up to date with the latest AI insights and innovations.
About the authors
Adam Kubany holds a PhD. in AI and has over 20 years of experience in IT. He specialises in leading and coaching research and development (R&D) teams to tackle complex Artificial Intelligence (AI) and Machine Learning (ML) challenges with responsibility.
Want to chat with Adam? Email adam@theoc.ai